Real-time Neural Radiance Caching for Path Tracing
Thomas Müller, Fabrice Rousselle, Jan Novák, Alexander Keller
Transaction on Graphics (Proceedings of SIGGRAPH 2021), vol. 40, no. 4
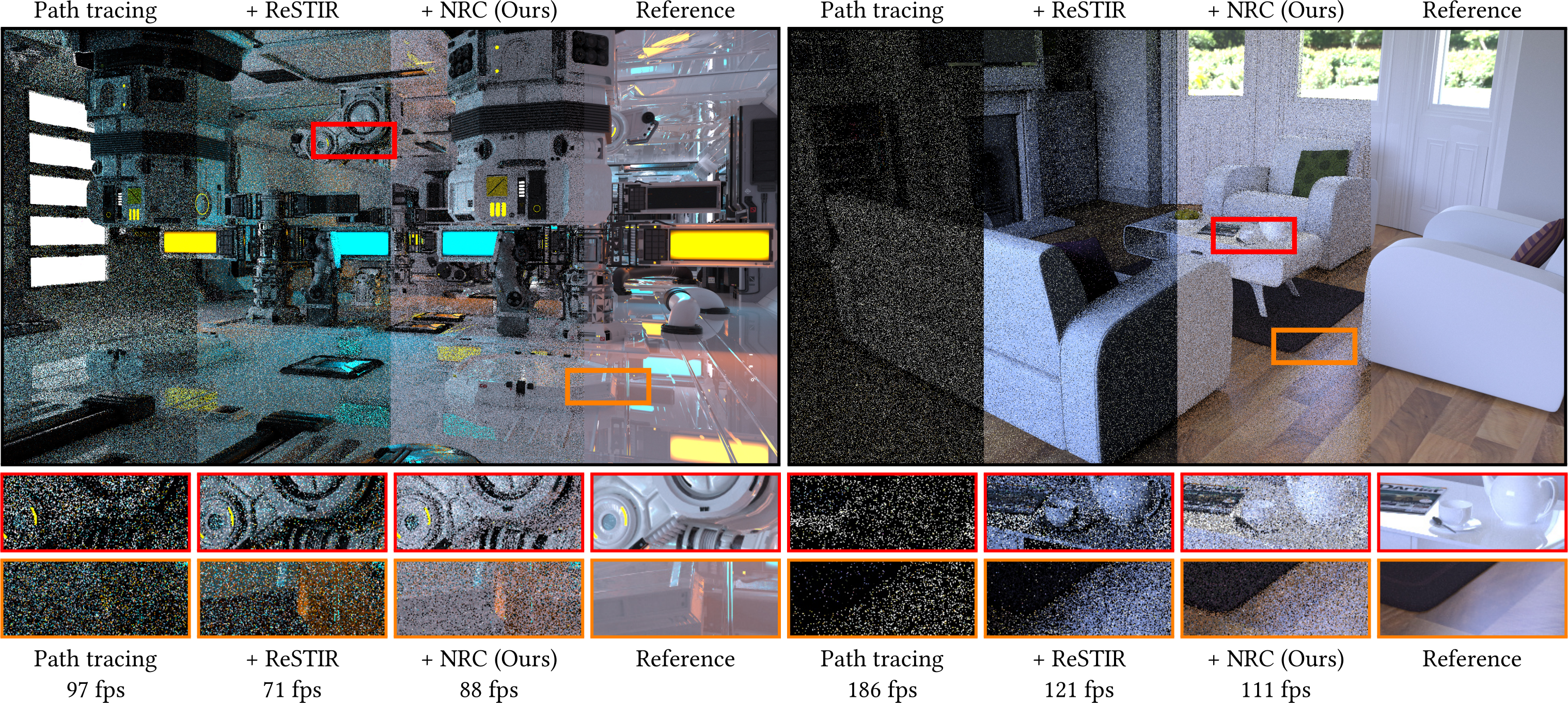
A path-traced frame from the Zero Day animation (left) and the Living Room scene (right), rendered with 1 sample per pixel each. Direct-illumination sampling such as ReSTIR [Bitterli et al. 2020] reduces noise at the first path vertex, but does not address noise from indirect illumination. We propose to reduce the remaining noise by (online) training a neural network to approximate the radiance field—a new take on classical radiance caching. Terminating the paths into the neural cache not only shortens paths—leading to an overall cost reduction in the Zero Day scene—but also removes most of the remaining noise while introducing little bias. The images were rendered at a resolution of 1920 × 1080 on a high-end desktop machine (i9 9900k and RTX 3090). Zero Day ©beeple
abstract
We present a real-time neural radiance caching method for path-traced global illumination. Our system is designed to handle fully dynamic scenes, and makes no assumptions about the lighting, geometry, and materials. The data-driven nature of our approach sidesteps many difficulties of caching algorithms, such as locating, interpolating, and updating cache points. Since pretraining neural networks to handle novel, dynamic scenes is a formidable generalization challenge, we do away with pretraining and instead achieve generalization via adaptation, i.e. we opt for training the radiance cache while rendering. We employ self-training to provide low-noise training targets and simulate infinite-bounce transport by merely iterating few-bounce training updates. The updates and cache queries incur a mild overhead—about 2.6ms on full HD resolution—thanks to a streaming implementation of the neural network that fully exploits modern hardware. We demonstrate significant noise reduction at the cost of little induced bias, and report state-of-the-art, real-time performance on a number of challenging scenarios.
downloads
publication
supplementals
video
presentation
citation
video
bibtex
@article{mueller2021, author = {M\"{u}ller, Thomas and Rousselle, Fabrice and Nov\'{a}k, Jan and Keller, Alexander}, title = {Real-time Neural Radiance Caching for Path Tracing}, journal = {ACM Trans. Graph.}, issue_date = {August 2021}, volume = {40}, number = {4}, month = aug, year = {2021}, pages = {36:1--36:16}, articleno = {36}, url = {https://doi.org/10.1145/3450626.3459812}, doi = {10.1145/3450626.3459812}, publisher = {ACM}, address = {New York, NY, USA}, keywords = {real-time, rendering, deep learning, neural networks, path tracing, radiance caching} }